How specialised AI delivers better returns on innovation
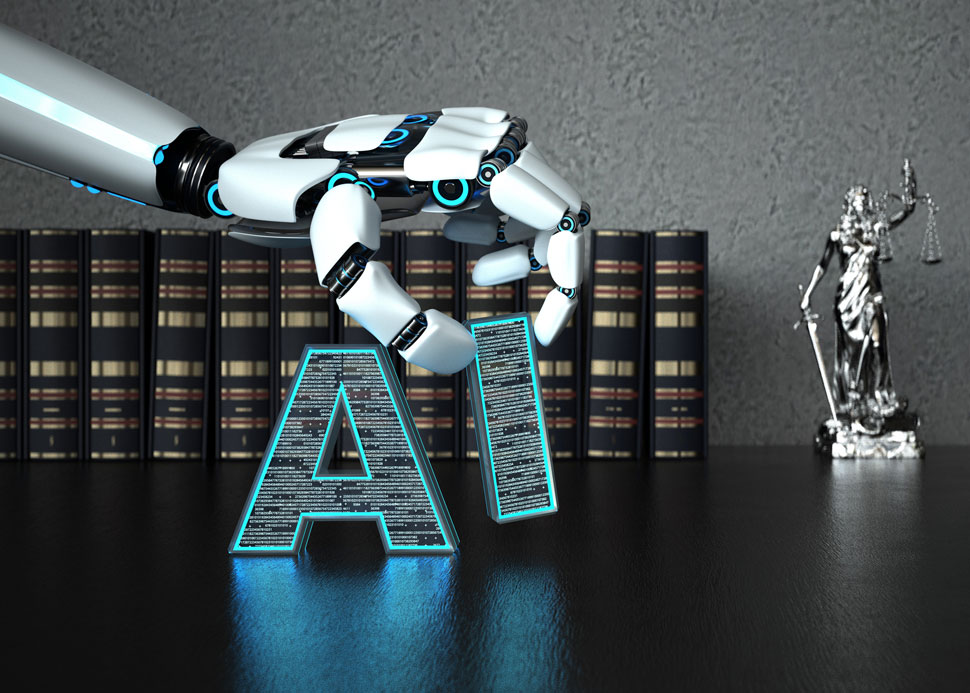
What do you think of when you think of AI? For decades, the public’s perception of AI has been of it as something science-fictional. Even as AI technologies got to work transforming how we interact with the world around us, as they have been for years, there was still a sense that ‘real’ AI was something that lay in our future.
The arrival of a new wave of generative AI changed all that, for two main reasons. First, open interfaces that anyone can use, tools like ChatGPT put the general public more closely in touch with the workings of AI, compared to something like a recommendation engine where the work happens behind the scenes. Second, and perhaps more importantly, these tools can attempt a broad, undefined range of tasks, not just those which their programmers have consciously built in. We might call these ‘generalist’ models – and that generalist nature gives them the capacity to surprise their users.
With this, assumptions about AI being a hypothetical future technology have subsided. Instead, the focus lies on ChatGPT, Midjourney, and their ilk of sometimes magical-seeming tools. It’s likely that you’re in a similar boat. But is this shift doing the technology full justice?
While generalist models are certainly impressive, there’s a case to be made that more significant business benefits will come from specialist models which offer a focused solution. For a good example of this, let’s turn to the field of law.
Data and privacy legislation like the General Data Protection Regulation (GDPR) and the California Consumer Privacy Act (CCPA) has given people new tools to take control of their data. As a result, many legal departments have been charged with a major additional set of tasks, not least of which has been a rising tide of Data Subject Access Requests (DSARs).
You might have heard about them during the recent dispute between NatWest and Nigel Farage, with the latter submitting a DSAR after his account at Coutts was allegedly closed due to his political values. When a business receives a DSAR, it has just 30 days to deliver an exhaustive package detailing all personal information it holds relating to the requester. The race is then on for in-house legal teams to assemble, sort, and analyse vast databases for any mention of the individual in question. The result? Weeks spent manually trawling through emails, text messages, Slack conversations and Excel spreadsheets.
For one business we recently worked with – UK-based technology company proSapient - responding to just one DSAR meant either committing tens of hours of precious legal resource to the problem, or else footing outsourcing fees which can run to £22,000 for one project alone.
DSAR projects feature a combination of high precision and repetitious workflow which makes them an ideal candidate for automation through a specialised AI model. Once the team adopted our solution, they were able to cull a dataset of 166,000 documents down to a body of 800 relevant pieces in just four hours. With AI capable of rapidly reading and filtering information, distinguishing relevant information from irrelevant across mountains of data, this meant that proSapient’s legal team was able to double its response speed and save over £20,000 in costs.
The capacity for generalist models to surprise us makes them superficially impressive, but also means that they will never create this kind of real-world value. Just take the recent example of the lawyers in New York who were fined for submitting fake case law invented by ChatGPT to court. In the legal world, as in the medical or financial spheres, where the minutiae of detail determines the outcome, generalist AI simply poses too much risk of inaccuracy or ‘hallucinations’.
This is not to diminish the importance of generative AI. It represents a breakthrough in computers being able to perceive language and images with human-like nuance and sensitivity, and that it also what makes the new generation of specialist AI models possible. In our DSAR example, for instance, that means being able to assess whether a piece of information buried fifty emails deep into a conversation relates to the requester or not – something that a simple search engine could never achieve.
There is, then, still a learning journey for assumptions about AI to go on. Far from being all-purpose aides, the AI tools that make a difference will be those built to answer specific problems efficiently and reliably. The businesses that realise this soonest have a real competitive advantage to win.